AI Hallucinations: Why They
Happen and How to Stop Them
Artificial
Intelligence (AI) has now become an
industry-disrupting technology in a few
industries, such as healthcare, finance, and
customer service. Nowadays, the business
industry employs
AI solutions for
automation, business intelligence, and customer
solutions. But even with its advancement, AI
faces a puzzling issue: the problem of
hallucination.
AI hallucinations are when
an AI system has produced incorrect or somewhat
misleading pieces of information and poses them
as facts. Such happenings can be hilarious in
ordinary chat but dangerous in serious
situations like consultations in law, medicine,
or finance. AI's reliability will be affected by
understanding how it makes and distinguishes
hallucinative reasoning .
How AI Develops
Hallucinations
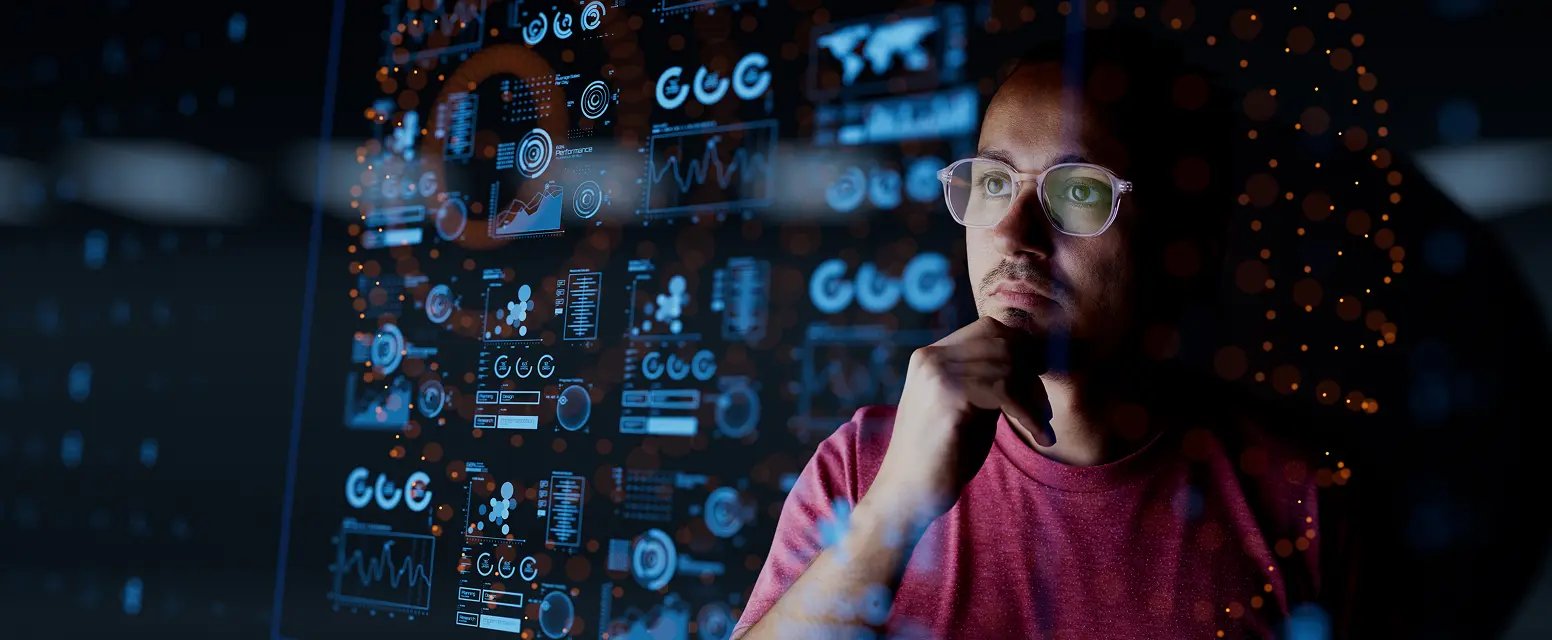
At its very core, AI identifies patterns within
massive datasets and generates an output based
on probabilistic models. Unfortunately, this
process has certain drawbacks. When AI
encounters gaps in knowledge or ambiguous input,
it attempts to fill in the blanks; sometimes
incorrectly. Unlike people, AI doesn't get
things or have gut feelings; it just guesses the
words that come next based on what it learned
before.
Hallucinations can cause due to several factors:
- Data
limitations: AI models are only as good as
the data used to train them. If the dataset
is incomplete or carries some bias, AI can
generate misleading responses.
- Overgeneralization:
AI has a tendency to continue from the past
pattern even when little information is
available which results in made-up outputs.
- Lack
of Real-Time Verification: Unlike humans who
can fact-check, AI lacks built-in mechanisms
to verify the accuracy of its responses
dynamically.
Grounding AI: Keeping It Real
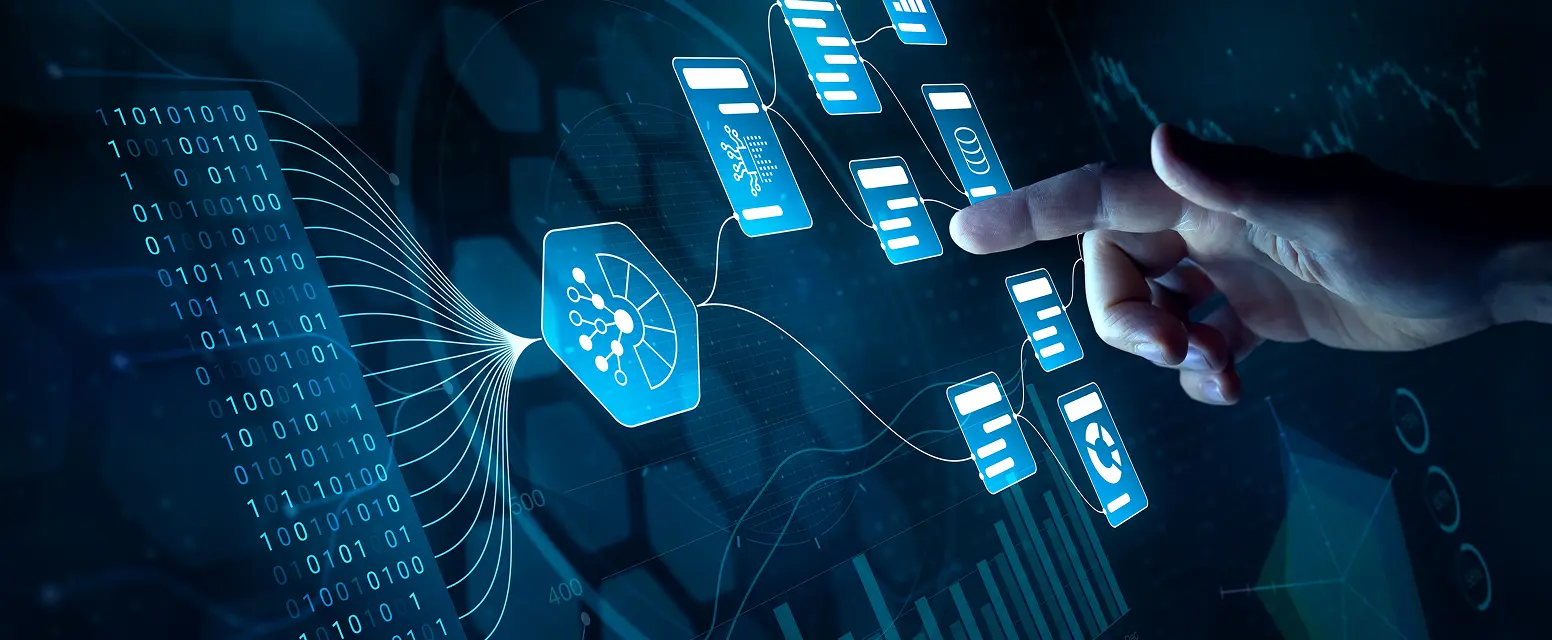
This would
imply trying to mitigate hallucinations through
a systematic process that will ensure the
responses given by AI are based on verifiable
information. This process is known as grounding,
which links AI-generated outputs to reliable
data sources.
1. Retrieval-Augmented
Generation (RAG)
RAG enables the AI models to be
improved such that they can locate real-time,
relevant information from some trusted source
before shaping their answers. This technique
lowers the chances of hallucination since it
allows for the integration of checked and
external knowledge instead of singularly relying
on pre-trained data.
How RAG works:
- The AI
receives a query.
- It
retrieves relevant information from curated
databases.
- It
synthesizes a response based on factual
data.
By incorporating external
verification, RAG helps AI remain accurate,
preventing it from "making things up."
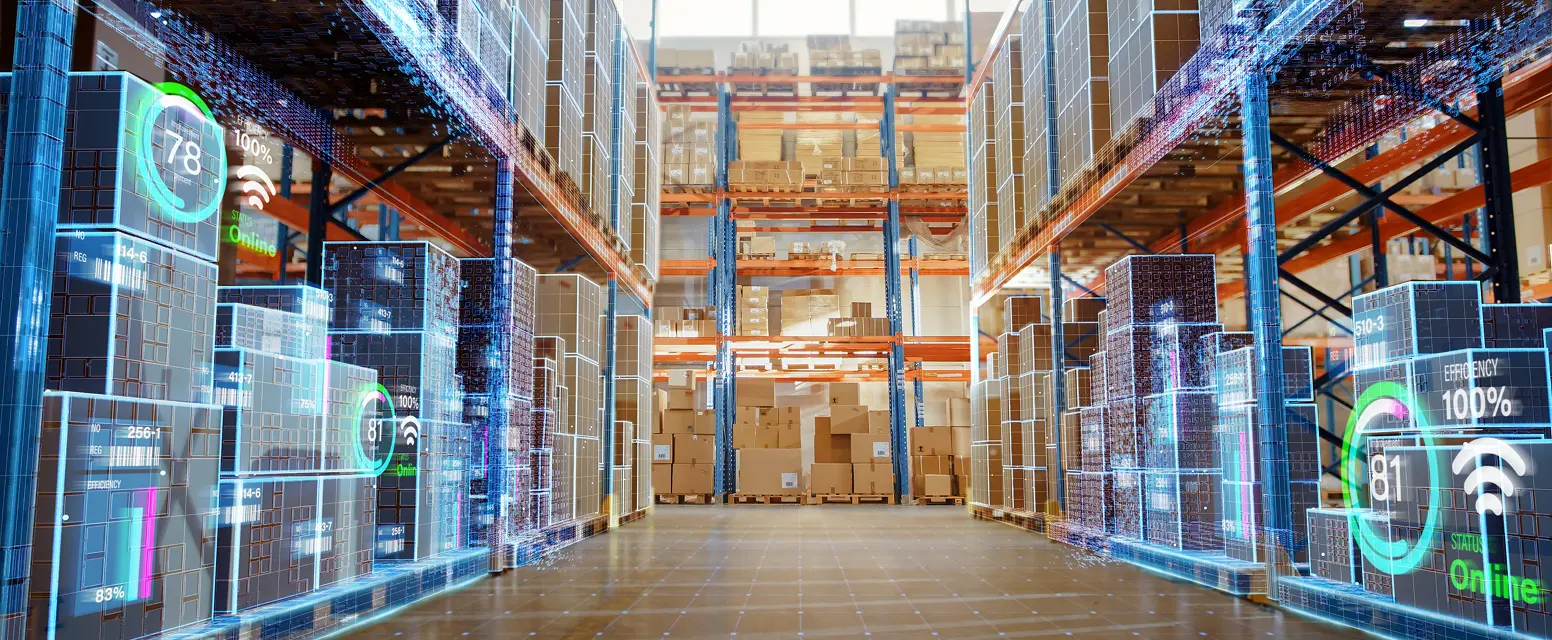
2. Prompt Engineering- The
Ability of Asking the Right Questions
By incorporating external
verification, RAG helps AI remain accurate,
preventing it from "making things up."
Best practices for prompt
engineering:
- Be Specific: Ambiguous
prompts lead to uncertain outputs. Instead
of "What's the return policy?", ask
something like, "Please provide the return
policy according to the official company
website."
- Provide Context: Providing
background information will help the AI be
more precise in its answer. For example,
"According to our 2024 company handbook."
This should help make it more accurate.
- Set Constraints: Limiting
AI's response scope prevents speculation.
Direct instructions like, "If you don't have
a verified answer, state that the
information is unavailable" help avoid
misinformation.
- Fallback mechanisms: AI
should realize when to tune back its
response because it does not have adequate
data to generate a response. It should
either ask for more context or indicate the
input of a human being.
The Business Impact of Grounding AI
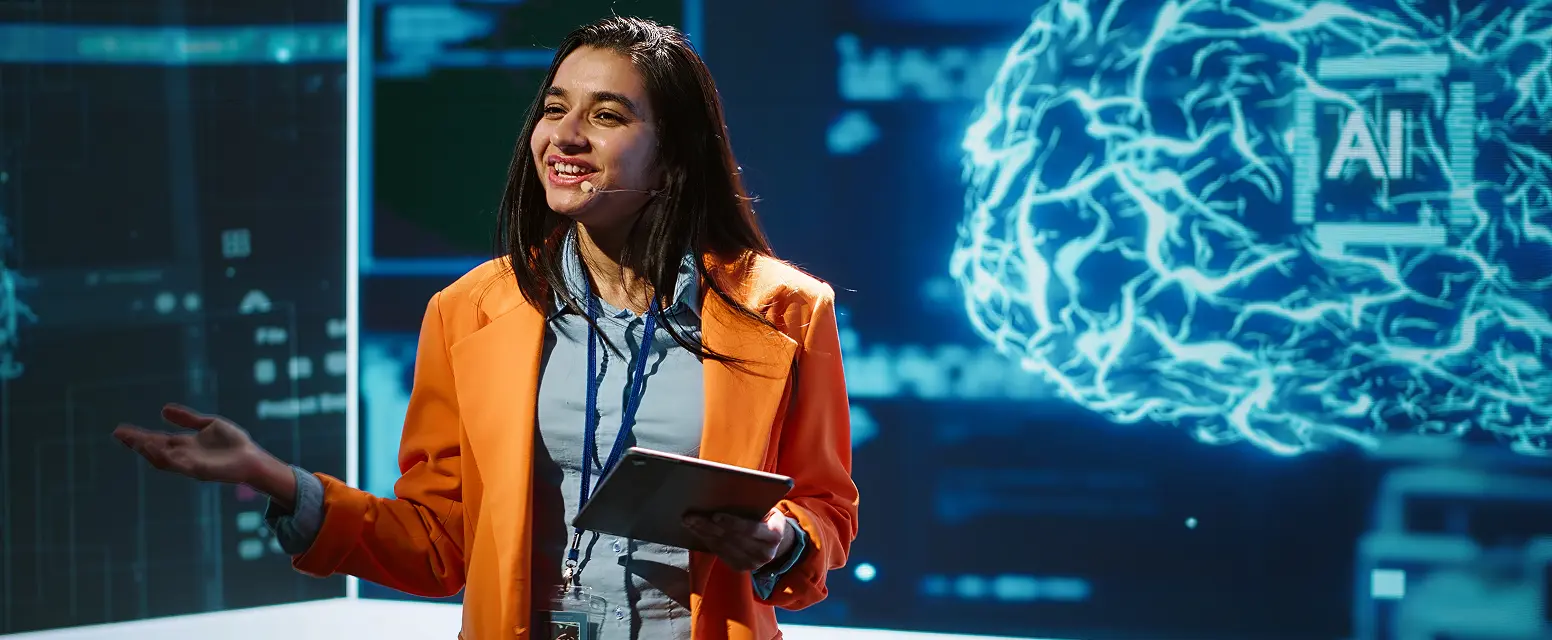
Assuring
the reliability of AI is no longer a technical
necessity but rather a business imperative.
Untrustworthy outputs from AI can undermine
consumer trust, damage brand reputation, and
create the potential for costly legal
ramifications. Organizations committing to
implement grounding techniques will be rewarded
by AI that is not only intelligent but also
reliable.
Studies
show that 65% of consumers tend to trust
organizations that are transparent about the use
of AI. By rolling out robust grounding methods,
the companies can build customer assurance and
command the moral leadership of AI deployment.
Looking Ahead: The Future of AI Accuracy
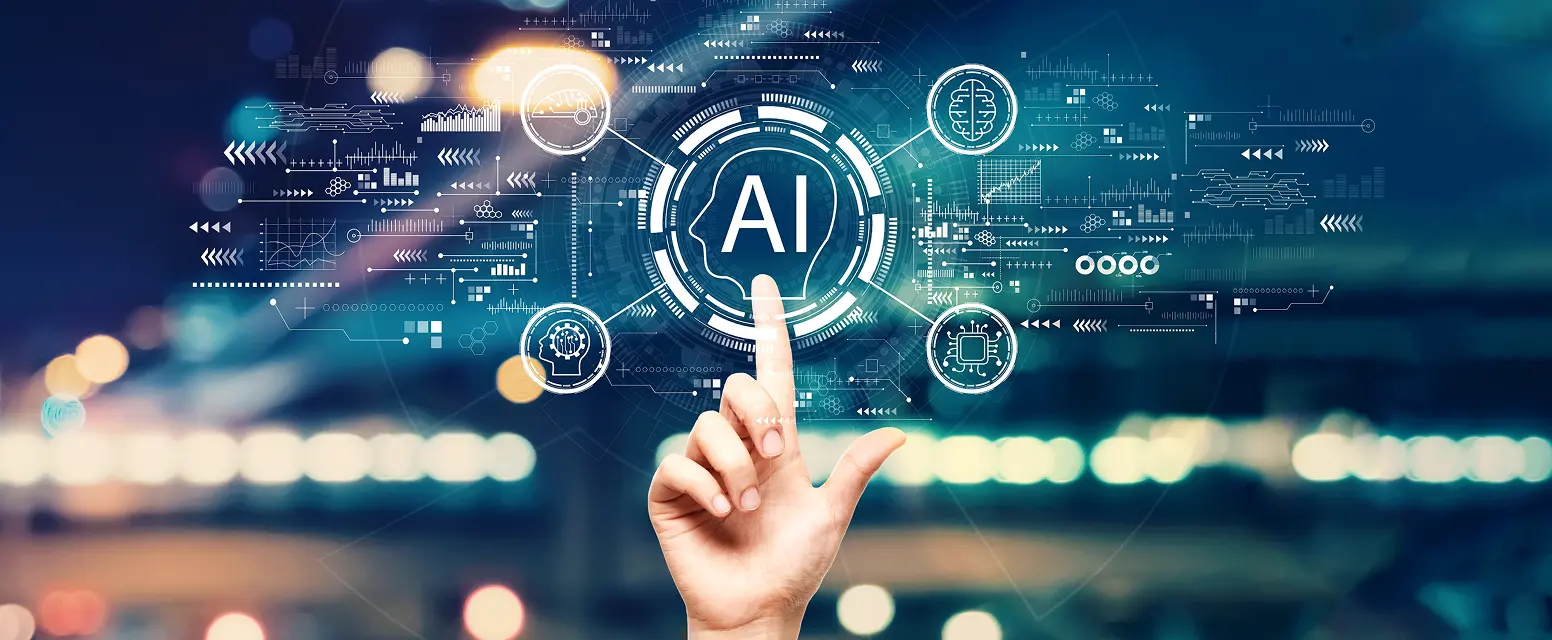
Though AI
hallucinations could not vanish overnight,
changing grounding approaches are leading us on
a more reliable route for artificial
intelligence systems. AI will become even more
reliable from continuous developments in
retrieval-augmented methods, real-time
verification, and quick feedback.
We're not
trying to limit AI's abilities; we just want to
make them better. With the right setup, AI can
be a helpful assistant that not only sounds good
but also provides accurate and trustworthy
information. The future of AI is about being
smart but also being right and responsible.